Timing of alfalfa cutting is a critical management practice to maximize yield potential, quality, and profitability. The University of Wisconsin, Purdue University, and Cornell University are developing a United States Department of Agriculture (USDA)-funded web program, Alfadvisor, which will provide alfalfa growers with economically optimum harvest recommendations. The platform will estimate alfalfa growth and quality changes, drying rates, and weather-related risks incurred during harvest. The online platform will provide easy-to-use tools to assess different cutting options and develop plans to best satisfy each grower's risk preferences.
A team of engineers, economists, and agronomists from the Midwest and Northeast are combining their expertise to develop this program, which will be available soon. This will be a free public cyber platform that combines satellite remote sensing, machine learning, and economic modeling to provide timely estimations of alfalfa yield and quality at the field scale. The program will also optimize harvest scheduling by integrating the trade-offs between alfalfa yield and quality, drying rate, and weather risks.
Satellite multispectral data has been used to estimate yields in various field crops, including corn, soybeans, and wheat. This is one of the first attempts to evaluate both yield and quality of a forage crop with multiple harvests in a season using satellite images. Different types of satellites (optical versus radar) collect different data at different time intervals. Data has been successfully combined from multiple satellite sources to improve alfalfa yield and quality predictions.
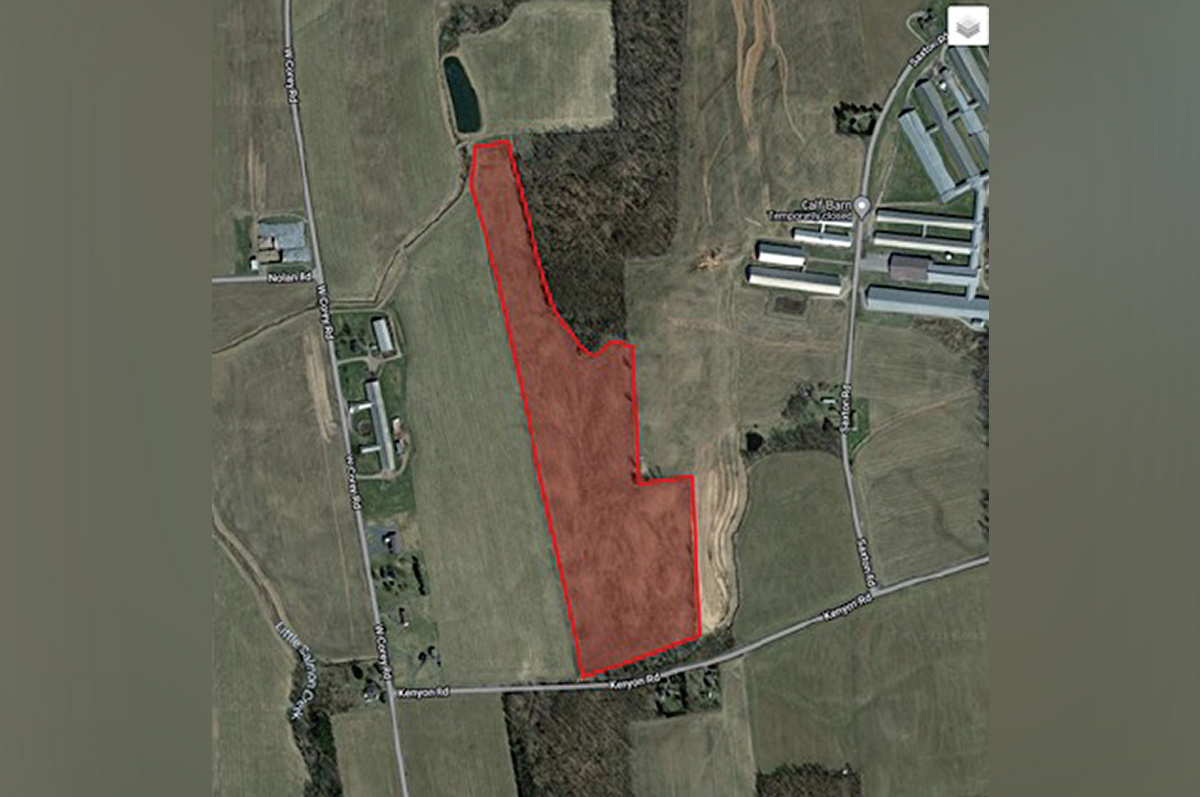
Alfalfa samples were collected in southern Wisconsin and central New York over the 2022 and 2023 growing seasons. Prior to each of four harvests during the season, there were four to five sampling dates with two- to four-day intervals between them. Five sites were sampled within a field on any given day, recording the GIS coordinates for each site. All samples were analyzed for yield and quality components. Sample data was used to calibrate the spectral data obtained from satellites.
A complex data fusion method was developed to combine data from different satellites with different spatial and temporal resolutions. Several machine learning models were compared for their utility to combine satellite images, environmental factors, and ground data to estimate alfalfa yield and quality traits. Alfalfa yield and quality traits were accurately predicted using fused multisource satellite data.
Alfalfa drying rate data was collected over several seasons using both automated and manual drying stands and used to develop drying rate curves under various types of weather conditions. A field boundary tool was developed to easily draw field boundaries on an interactive map. Once a field boundary is established, NOAA weather forecast data is automatically downloaded for the specific site.
From an economic perspective, some preferences will be requested from the user, such as type of storage, intended forage use, or harvest urgency. Drying times will be estimated along with the probability of rain exceeding a threshold during the drying period. Weather forecast data, along with yield and quality models and drying rate models, are combined to provide a set of harvest options with detailed expectations of quality and risk.
One potential issue with using satellite data surfaced in 2023. Canadian wildfire smoke engulfed the sampling area over a couple of weeks, which can affect satellite images. Satellites can measure aerosol optical depth, which quantifies the effect of smoke particles in the air. This effect can then be subtracted from the satellite surface reflectance data, eliminating the problem.
Although initially developed for pure alfalfa stands, the program may also be sufficiently accurate for estimating yield and quality in stands with a modest amount of grass present. Harvest of mixed stands mostly consisting of alfalfa is typically based on alfalfa maturity/quality. A mixed stand with up to 20 percent grass on a dry weight basis may only have three to five percent grass visible from the top of the canopy. For these fields, satellite scans should essentially be seeing/analyzing pure alfalfa.
AlfAdvisor is farm-size neutral; all that is needed is a computer and at least one alfalfa field. This program has the potential to replace the numerous “scissors-cut” projects for estimating alfalfa quality over a region by providing instantaneous quality estimates for a specific field. Initially, model parameters for the program will be specific to the Midwest and Northeast, with the potential to expand nationwide.
This project was funded by USDA’s Alfalfa Seed and Alfalfa Forage Systems program.
Jerry Cherney (Cornell University), Zhou Zhang (University of Wisconsin), Jinha Jung (Purdue University), Paul Mitchell (University of Wisconsin), and Matthew Digman (University of Wisconsin) are AlfAdvisor collaborators.
![]() |
This article appeared in PRO-DAIRY's The Manager in March 2024. To learn more about Cornell CALS PRO-DAIRY, visit PRO-DAIRY. |